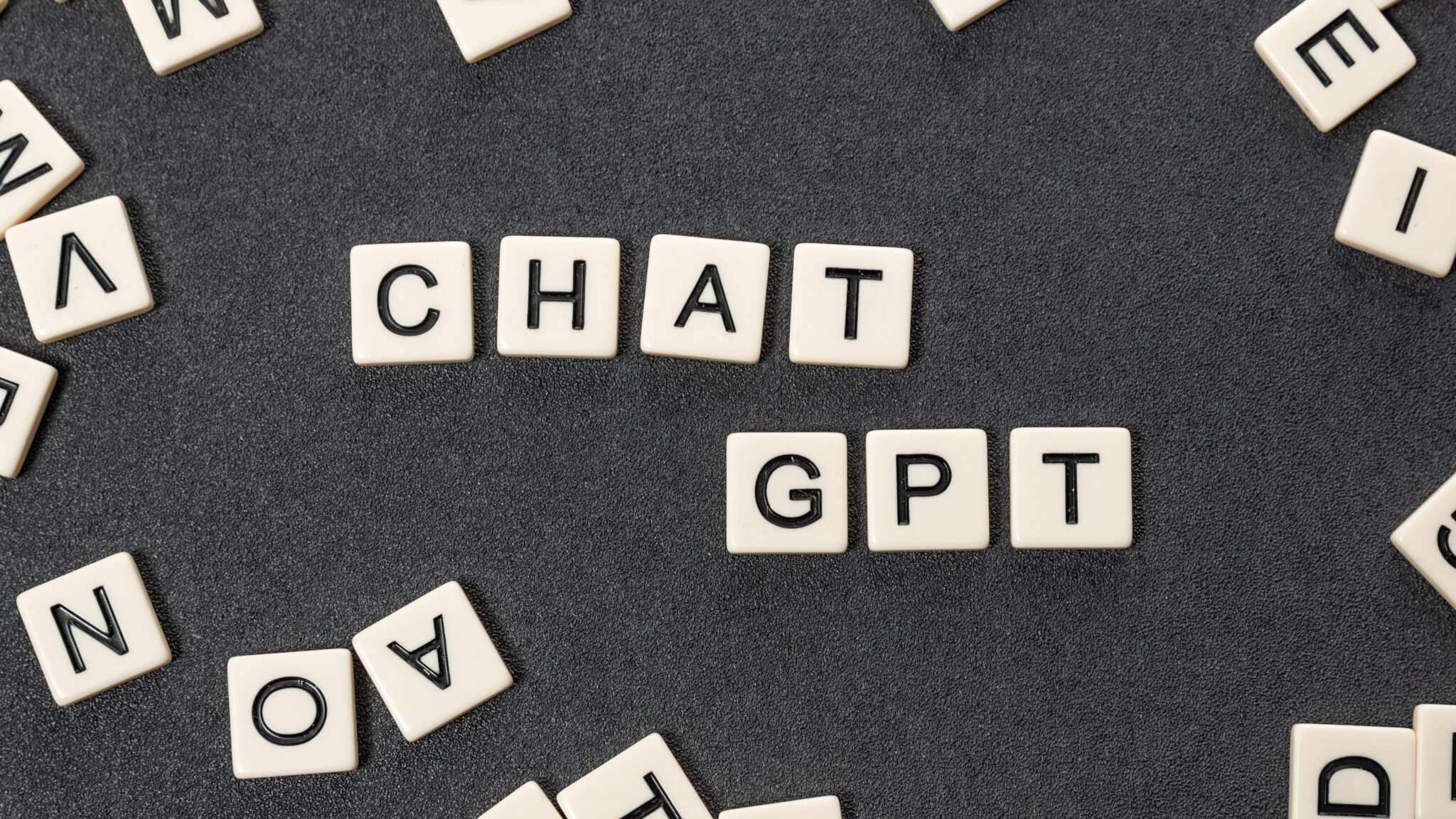
Fighting fraud in the age of ChatGPT.
Large language models (LLMs) like ChatGPT are making it easier than ever for criminals around the world to scale and customize their fraud.
To cope with the rise in suspicious transactions that these technologies will create, banks need solutions that can respond faster and smarter.
As traditional fraud and anti-money laundering transaction monitoring systems have improved during the past 10 years, criminals have professionalized their skills to scam people and perpetrate identity fraud. Generative artificial intelligence takes this to the next level.
These language models are already being used to create highly convincing text, from phishing conversations to fake invoices, contracts, and financial statements. Law enforcement agencies forecast a “grim outlook” as criminals exploit LLMs in increasingly sophisticated financial crimes.
“Solutions like ChatGPT have the potential to help fraudsters in mass customizing their communications with victims, making the scale of possible vulnerable individuals substantially larger and more difficult to identify,” says Sjoerd Slot, Sygno CEO. “While such providers will also need to monitor potential misuse of the technology, banks will need to see this as yet another technological fraud advancement. Static monitoring has long gone, change is the norm.”
The mainstreaming of AI is a yet another step in fraudsters advancement
The rapid growth in awareness about LLMs has been unprecedented. A wave of publicity following OpenAI’s public release of ChatGPT in November 2022 saw it gain more than 100 million users in just two months, setting a record for a consumer application. Many others have followed suit, like Google’s Bard and Meta’s LLaMA, leading to widespread use (and mis-use) of the technology.
It may already be too late to put up guardrails. The leak of Meta’s LLaMA models in March 2023 led to the release of compact open-source LLMs that can run on a laptop.
In the age of mass-market AI, traditional transaction monitoring approaches based on complex business rules will fall even further behind, increasing either the workload on compliance teams or the amount of undetected fraud – most probably both.
Instead of looking for ever-evolving suspicious behaviors directly, Sygno’s approach uses automated machine learning to train models to recognize legitimate transaction data. This type of behavioral detection is far more granular and and accurate as it is based on actual client behavior vs extrapolations of criminal activity. This can reduce the number of false positives that need to be reviewed, increase the number of true positives that are detected, and ultimately save time, money, and resources.