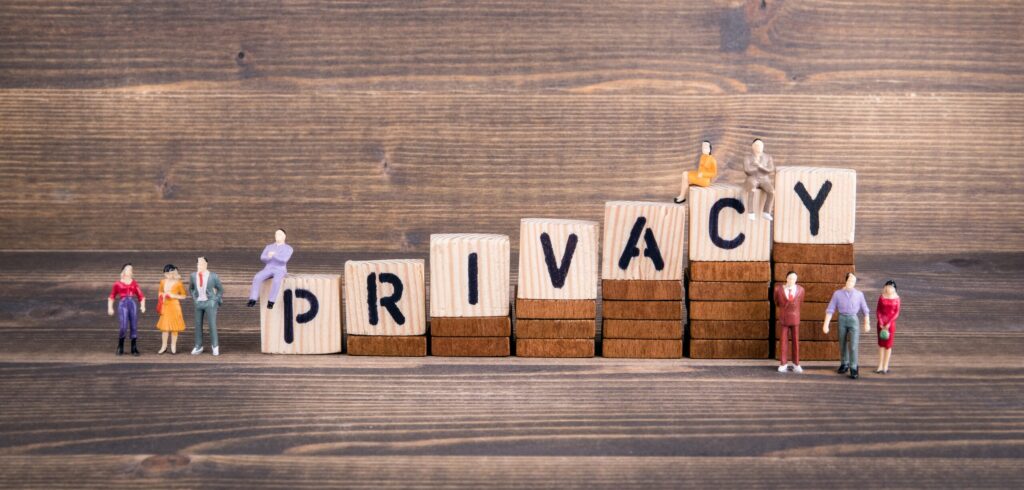
The Goldilocks algorithm: Detecting anomalies while respecting privacy rules
Transaction monitoring systems that generate excessive false positives lead to unnecessary invasion of privacy.
Striking a balance between data protection and anti-money laundering can be a challenge. Like Goldilocks, regulators want financial institutions to get it just right. They shouldn’t go on a fishing expedition and pry into customer data too much, but they also shouldn’t do too little and risk missing financial crime.
In the EU, for example, AML rules require financial institutions to implement systems that “enable the detection of unusual or suspicious transactions”, while GDPR requires data controllers to demonstrate a “legitimate interest” for processing personal data. Getting the balance right demands that FIs adopt an efficient approach to transaction monitoring.
Systems that generate excessive false positives are at risk of breaching GDPR if they lead to frequent investigations that require FIs to comb through innocent customers’ data. Hiding behind AML rules may not be a sufficient defense, as financial regulators also take a dim view of transaction monitoring systems that generate excessive false positives. There is a clear need to fight financial crime, but there is always a tension between privacy and AML provisions, as we have seen in debates around public registers of companies’ beneficial owners, sharing information, and so on.
So how should FIs deal with this tension – minimizing privacy impact while also flagging unusual and suspicious transactions?
When done right, anomaly detection algorithms result in fewer false positives and therefore fewer investigations. As a result, FIs can reduce the amount of personal data they process and lower their impact on the privacy of their valued customers. And that means we can spend more time on the risks within our portfolio.
At the same time, fewer false positives also improve our ability to detect financial crime and therefore save resources.
Getting started with detecting anomalies while respecting privacy rules
Get in touch with us to discuss how automated machine learning can help to generate anomaly detection algorithms that are just right.